
The integration of Automated Vehicles (AVs) into traffic systems offers significant potential to improve traffic congestion and efficiency. Our recent work introduces an adaptive speed controller designed for mixed autonomy scenarios, where AVs and human-driven vehicles interact.
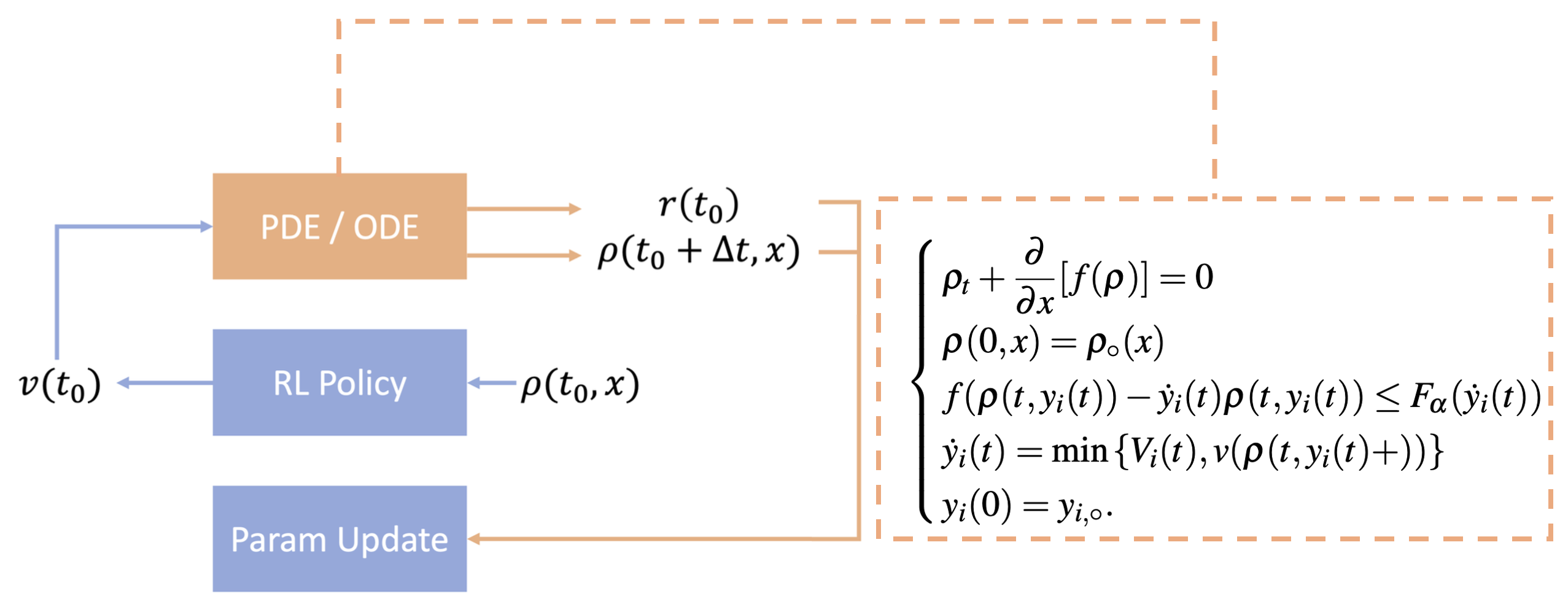
This study models traffic dynamics using a system of coupled Partial Differential Equations (PDE) and Ordinary Differential Equations (ODE). The PDE captures the flow of human-driven traffic, while the ODE characterizes the trajectories of AVs. A speed policy for AVs is derived using a Reinforcement Learning (RL) algorithm within an Actor-Critic framework, seamlessly integrating with the PDE-ODE model to optimize the AV control policy.
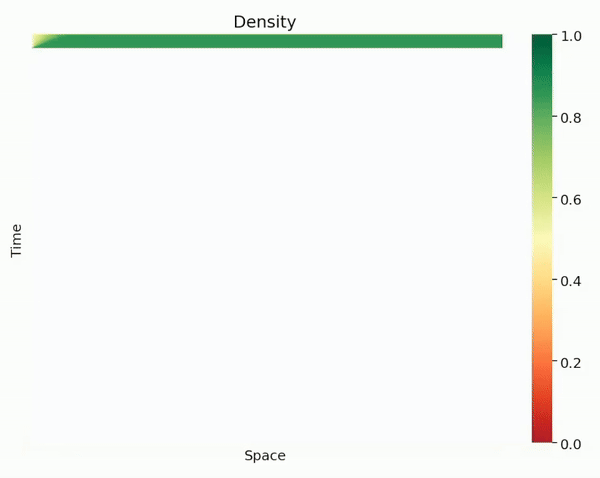
The RL-based adaptive speed controller was tested through numerical simulations, demonstrating improvements in key traffic metrics such as minimum flux, average speed, and speed deviation. These improvements highlight the potential of AVs to act as dynamic actuators within traffic flow, helping to mitigate congestion and enhance overall traffic efficiency.
Our approach frames the control problem within a Markov Decision Process (MDP) and employs the Actor-Critic method to update policy parameters. The adaptive controller's performance was evaluated in various traffic scenarios, showcasing its capability to adjust AV speeds in real-time and optimize traffic conditions.
The results of our simulations indicate that the adaptive speed controller can enhance traffic flow, reduce bottlenecks, and smooth traffic waves. The Proximal Policy Optimization (PPO) algorithm, used for optimizing the control strategy, showed significant improvements in traffic management through iterative refinement of AV control policies.
This research contributes to the theoretical understanding of traffic dynamics and provides a practical framework for deploying AVs to improve traffic systems. Future work will focus on expanding the scalability of this method, integrating real-world traffic data, and exploring the socio-economic impacts of AV-based traffic control systems.