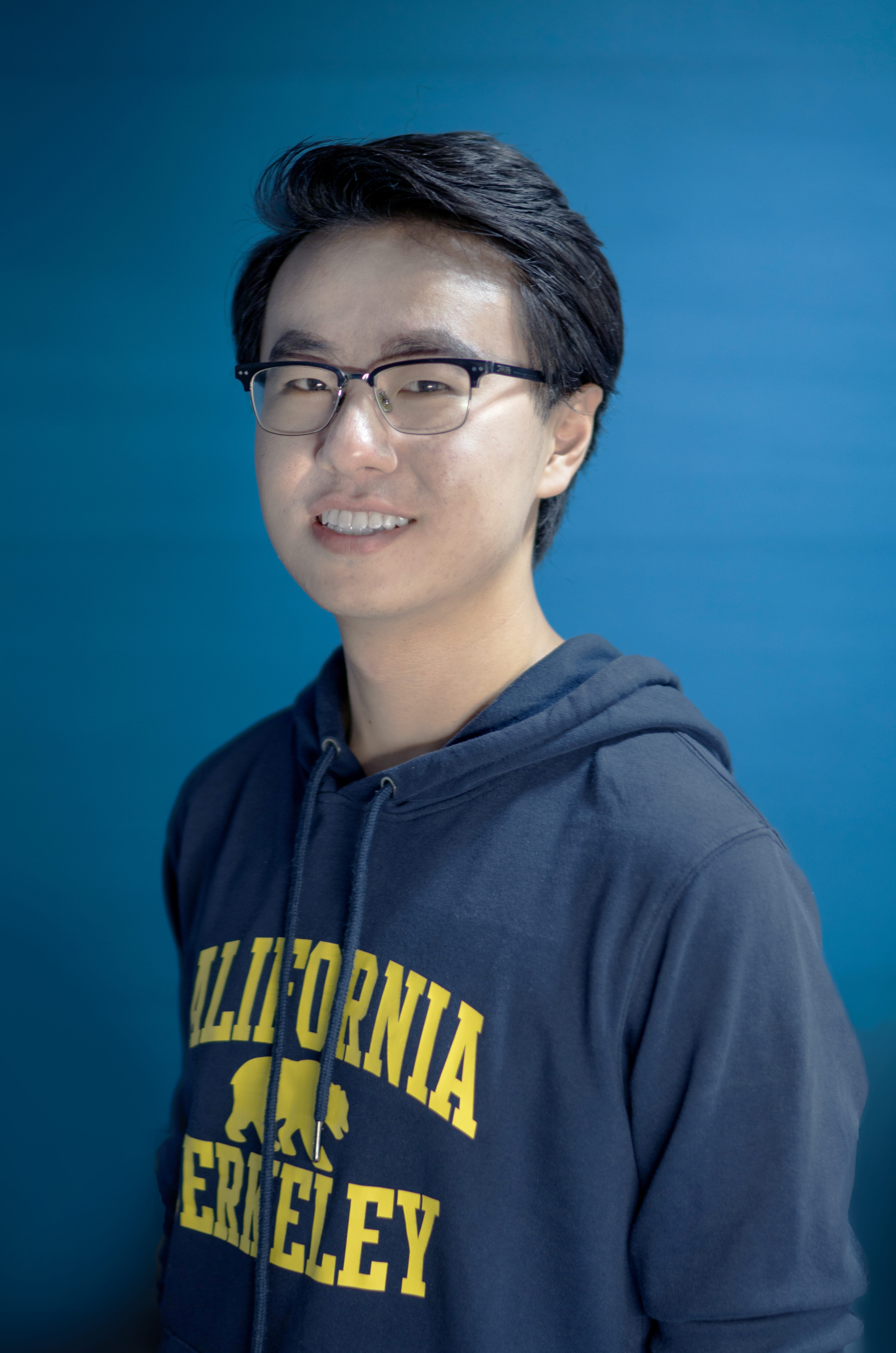
Welcome! I'm Han Wang, a Ph.D. candidate in Berkeley AI Research(BAIR) and Berkeley Deep Drive(BDD) at UC Berkeley. My doctoral research focuses on improving traffic flow and urban mobility with connected and automated vehicles using multi-agent system framework. I have an interdisciplinary background in Computer Science and Transportation Engineering, with a keen interest in vision science and generative AI.
I've worked on exciting projects around automated vehicles like developing speed planner for 100 AVs fieldtest, predicting vehicle trajectories and collision risk evaluations, supported by organizations including General Motors, Nissan, Toyota and Allstate. For personal projects, I enjoy working with AR/VR + GenAI technology and currently addicted to the development of Vision Pro.
Hope you find them as exciting as I do!